Feb 22, 2021
Marketing data warehousing 101: the ultimate guide for marketers and analysts
24-MINUTE READ | By Riku Mikkonen
[ Updated Jun 25, 2024 ]
You know how your marketing data lives across dozens of different platforms, including — but definitely not limited to — Google Analytics, Facebook Ads, LinkedIn, Google Analytics, and/or Salesforce?
A cloud-based marketing data warehouse (like Google BigQuery, Snowflake, or Azure Synapse Analytics) allows you to centralize all that data into a single destination that’s built for not only storing data but also analyzing it.
After reading this guide, you’ll know everything you need to know about marketing data warehousing, including:
- What is a marketing data warehouse?
- Data warehouses vs. databases vs. data lakes vs. data marts
- The main benefits of data warehousing for marketing and analytics teams
- Possible drawbacks of data warehousing for marketing and analytics teams
- When to start moving your marketing data into a warehouse
- How to choose the right data warehouse for your business
- How to get started with marketing data warehousing
1. What is a marketing data warehouse?
A marketing data warehouse is a cloud-based destination for storing and analyzing cross-channel marketing data. Data warehouses allow marketing and analytics teams to consolidate data from multiple platforms including advertising channels like Facebook and Google, web analytics platforms like Google Analytics, and CRM systems like HubSpot and Salesforce, among others.
Compared to alternative data storage and analytics solutions, the main benefits of data warehouses in the marketing context include relatively inexpensive and elastic storage as well as the ability to centralize data from multiple sources for more convenient analysis.
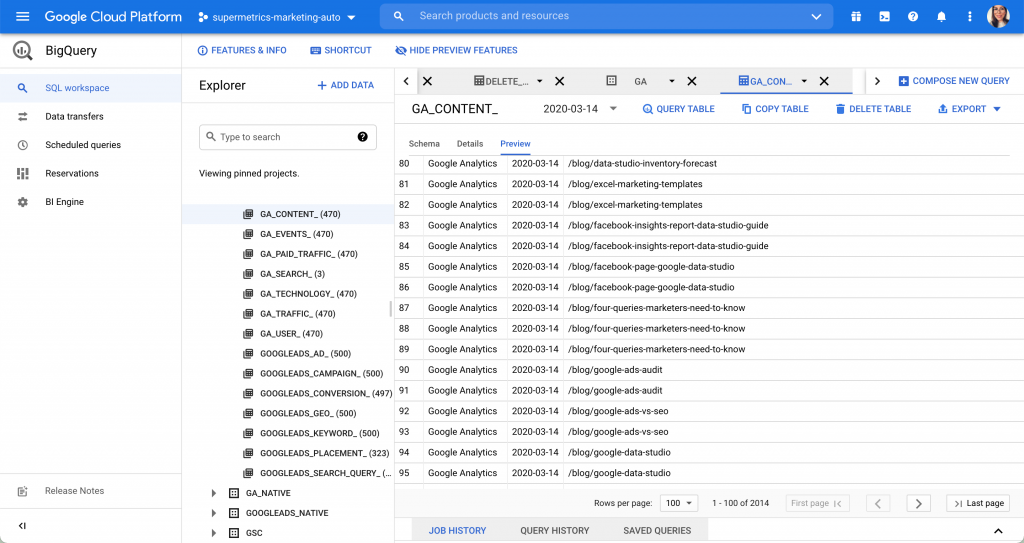
Data warehouses consist of structured tables, which makes it quick and easy to query the exact data you want to include in your report or analysis. Most data warehouses use SQL as the query language (but more on that a little later).
Data warehouses consist of two main elements:
Storage
Data warehouses allow you to store, centralize, and integrate granular data from multiple sources over a long period of time. This means that you no longer have to rely on your marketing platforms’ unique data retention policies. Instead, all the cross-channel data you need for historical analysis and benchmarking is stored neatly in one place — and for a relatively low cost. Your storage capability will grow as your dataset grows.
Computing
In addition to storage, data warehouses also support processing large amounts of data. With on-premise solutions, you can’t just provision more hardware to crunch your data. With a cloud data warehouse, this can be done with just a few clicks. This is critical for analytics, as you’ll want to be able to quickly query specific datasets.
To learn more about the basics of marketing data warehousing, check out our on-demand webinar, where our friend Khrystyna Grynko from Better&Stronger discusses getting started with data warehousing with a focus on Google BigQuery.
2. Data warehouses vs. databases vs. data lakes vs. data marts
The ugly truth is that most marketing teams still rely on simple spreadsheets and dashboard tools for data “storage”, analysis, visualization, and reporting. And while that’s all well and good with smaller data volumes, self-service analytics tools like spreadsheets and dashboards won’t be able to handle your ever-growing quantity of marketing data.
The good news is that sturdier data platforms — like data warehouses, databases, data lakes, and data marts — are quickly increasing in popularity.
But what are the differences between these different technologies? Let’s take a quick look.
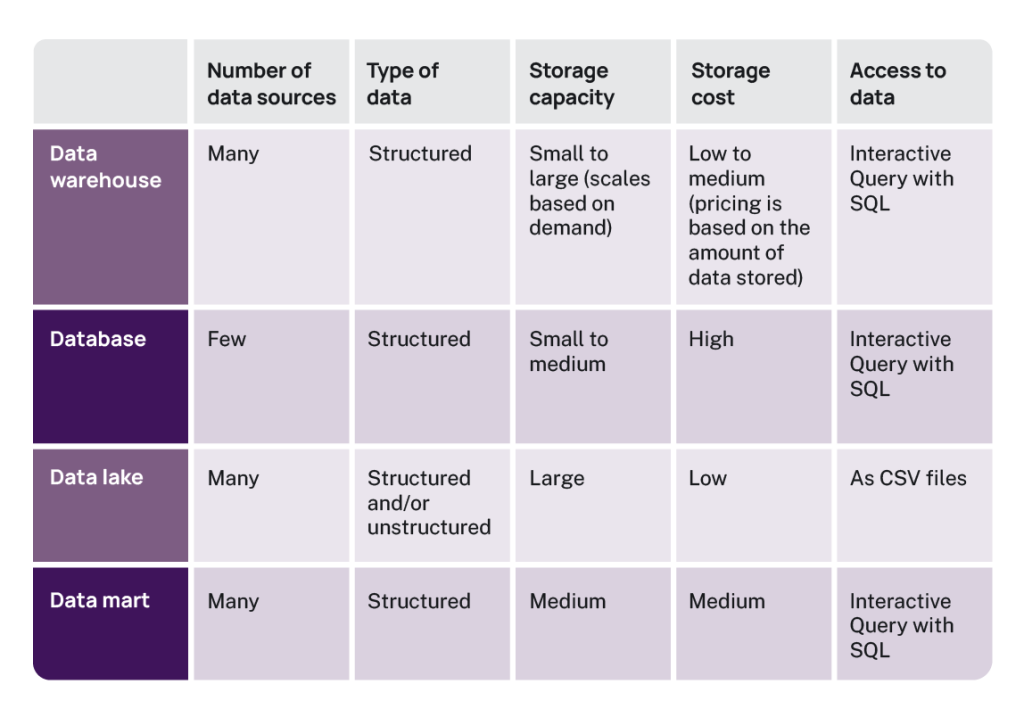
Data warehouse
A data warehouse is a cloud-based platform that allows you to store and analyze structured cross-channel and cross-department data. A data warehouse consists of tables and typically uses SQL as the query language.
- Number of data sources: Many
- Type of data: Structured
- Storage capacity: Small to large
- Storage cost: Low to medium
- Access to data: Interactive Query with SQL
Database
A database is a highly organized collection of structured data that’s easy to access, manage, and update. A single database typically only contains mutually related data. For example, your CRM is a database used for storing information about your customers and prospects.
- Number of data sources: Few
- Type of data: Structured
- Storage capacity: Small to medium
- Storage cost: High
- Access to data: Interactive Query with SQL
Data lake
A data lake is a central repository of data that allows you to store data in its raw format, whether that’s structured or unstructured. You can think of a data lake as your Google Drive folder, where you store a mix of images, spreadsheets, videos, audio files, and text docs.
- Number of data sources: Many
- Type of data: Unstructured and structured
- Storage capacity: Large
- Storage cost: Low
- Access to data: As CSV files
Data mart
A data mart is a subset of a data warehouse that typically serves a specific business line. A “marketing data warehouse” can, in fact, be a data mart if you’re also storing other departments’ data in the same data warehouse instance.
- Number of data sources: Many
- Type of data: Structured
- Storage capacity: Medium
- Storage cost: Medium
- Access to data: Interactive Query with SQL
To sum up, if you’re looking for long-term storage for all your marketing data, you basically have two options:
- A data mart / a data warehouse for storing marketing data in a structured format (The main benefit here is that structured data is a lot easier to query and analyze than unstructured data.)
- A data lake for storing your marketing data in a structured and unstructured format (The main benefit here is that you can store raw data in a wide variety of formats, including images, videos, text files, etc.)
But speaking of benefits, let’s discuss the main advantages of data warehouses.
3. The benefits of data warehousing for marketing and analytics teams
The major benefits of using a cloud-based marketing data warehouse include:
A. Creating a single source of truth
You know how scattered marketing data has a tendency to slow marketing teams down?
That’s because either marketers simply don’t have the time to sign in to a dozen different platforms to collect the data they need to make better decisions or they waste so much of their time on data collection that they don’t have time for analysis and optimization.
Either way, data warehouses alleviate those pains by consolidating all the data to create a single source of truth. This helps marketers get a better handle on important metrics like customer acquisition cost (CAC), return on investment (ROI), return on ad spend (ROAS).
B. Time to insight
You don’t need to provision expensive hardware or get access to a physical data center to start centralizing your marketing data in a cloud-based warehouse. Simply choose your data warehouse (e.g. Google BigQuery, Azure Synapse Analytics, or Snowflake) and start moving your data with a fully managed pipeline like Supermetrics.
And since getting started only takes a few clicks, you can immediately start pulling insights from your DWH.
C. Analytics capabilities
Whether you want to query data with SQL or feed data from your data warehouse directly into a data visualization or BI tool, your data warehouse can process complex queries in seconds and further push the data you need into your reporting and/or analytics tools of choice. Key analytics tools, such as Looker Studio (formerly Google Data Studio), Power BI, and Tableau, can pull real-time data from your data warehouse instance without extra configuration.
D. Full ownership of historical data
Instead of depending on data retention policies of Facebook, Google, HubSpot, and your other platforms, data warehouses allow you to securely store your cross-channel data in one place.
With full ownership of and access to all your historical marketing data, you’ll be able to pull the exact datasets you need to make better decisions about the future.
E. Inexpensive and elastic storage with low to no maintenance needs
Whether you work for a growing SMB or an enterprise company, storing marketing data in a cloud-based data warehouse is relatively cheap. With elastic storage, you’ll never run out of capacity, as your data warehouse grows with your business.
Cloud-based marketing data warehouses are also known to require low to no maintenance since the cloud provider takes care of the upkeep for you. You just pay for the resources you use.
4. Possible drawbacks of data warehousing for marketing and analytics teams
Even though the benefits of data warehousing are hard to argue with, we’re not trying to say that everyone and their grandma should jump on the DWH bandwagon just yet.
And while there aren’t many downsides to data warehousing on an organizational level, you might want to consider the impact the technology will have on your marketing and analytics teams.
A. Possible downsides for marketing teams
While fully managed marketing data pipelines like Supermetrics make it easy to move data into the warehouse itself, the problem is that there aren’t many marketers who know how to write SQL queries to get any meaningful data out of the warehouse.
Without knowing how to write SQL, you won’t make the most of the flexibility and power of a data warehouse. Nevertheless, simply using it for intermediate storage of your data for use in your analytics, reporting, data visualization, or BI tool of choice will already give performance benefits.
This might, of course, make your marketing team more dependent on your analytics team for insights and reports. Alternatively, you can always invest in upskilling your marketing team and providing them with SQL training.
B. Possible downsides for analytics teams
On the other hand, suddenly having your marketing team rely on your analytics team for reporting and ad hoc data crunching may also reflect negatively on your analytics team’s productivity.
If, for example, your marketing team has been using self-serve analytics tools like spreadsheets or data visualization platforms and can no longer access data on their own, the analytics team may become a bottleneck for decision making.
That’s why, before you invest in a marketing data warehouse, it’s a good idea to come up with the rules of engagement between the two teams. Once you have a good idea of the division of labor and the work expected from both sides, you’re in a better position to implement a marketing data warehouse.
Speaking of implementation, let’s take a look at some of the telltale signs that now might, in fact, be the right time to start moving your marketing data to a warehouse.
5. When should you start storing your data in a warehouse?
While there probably are many right answers to this question, I’m going to serve you with two simple ones:
- Your business — and data volume — are growing
- You need/want to better understand the return on marketing investment across different channels, campaigns, and tactics
Let’s look at each of these in turn:
A. Your business is growing in size and/or complexity
Here at Supermetrics, we started using Google BigQuery as our marketing data warehouse right around the time we were getting ready to launch a new product: Supermetrics for BigQuery (pretty meta, right?).
For us, introducing our eighth product meant that we were going to be swimming in data. And while we had somehow managed to do most of our marketing reporting and analytics in spreadsheets and Google Data Studio until then, we simply knew that they were no longer going to cut it.
Along with the new product, we rolled out a new sales-assisted go-to-market strategy. And while we’d previously lived and breathed the self-serve SaaS model, we suddenly had two completely separate funnels to track: the established self-serve funnel and a brand new sales-assisted one.
Needless to say, this new structure added complexity to our data analysis and reporting, essentially doubling the number of KPIs we needed to track on a daily basis.
In other words, thanks to our new business model, we outgrew our simple marketing data stack.
Similarly, many of our customers have transitioned into marketing data warehousing when they’ve experienced one or more of the following growing pains:
- Slowly loading — or crashing — spreadsheets (or otherwise insufficient analytics tools)
- A fundamental change in the business model (e.g. from transactional sales to subscription sales)
- Multiple stakeholders needing access to data and insights
- A growing ad budget necessitating a better understanding of ROAS
- Rapid business growth (which also means a rapid growth in the amount of data)
To perfectly illustrate the last point, our customer Sebastian Mehldau from fast-growing e-bike company VanMoof says:
“The more campaigns we ran and the more customers we had, the more complex things became. At the pace we were growing, we just couldn’t work like that. We desperately needed to centralize our data.”
Sebastian Mehldau, Growth Marketing Manager, VanMoof
To hear more about VanMoof’s data warehousing journey, check out this on-demand webinar where Sebastian shares his best pieces of advice for running successful data warehouse projects from start to finish.
B. The need to better understand marketing ROI, ROAS, and attribution
The other common reason to start moving marketing data to a warehouse is an increasing need to prove the value of marketing.
For example, this can happen as a result of:
- A change in marketing/business leadership
- Growing pressure from the board of directors and/or investors
- An economic downturn and/or other unfavorable changes in market conditions
For example, when COVID 19 first hit globally in the beginning of 2020, many of our SMB customers suddenly needed to tighten their budgets to make sure they could weather the unpredictable business impacts of a global pandemic.
However, their goal wasn’t to stop doing marketing altogether but to allocate resources into the channels and campaigns with the highest potential return.
As a result, during 2020, many of our customers and prospects decided to invest in a single source of truth into their marketing spend and returns. In most cases, the solution was setting up a cloud-based marketing data warehouse in BigQuery or Snowflake.
Long story short, if you’re experiencing any of the following symptoms, it may be time to invest in a marketing data warehouse:
- Unfavorable market conditions making it necessary to “cut the fat”
- A need to better understand marketing ROI
- The aspiration to get started with attribution modeling, predictive marketing analytics, or marketing mix modeling
Psst! For more insights into the varied reasons behind our customers’ data warehouse projects, make sure to check out our customer success stories.
6. How to choose the right (marketing) data warehouse for your business
The most common data warehouse solutions for marketing include:
- Google BigQuery: Due to its native integrations to Google’s own platforms like Google Analytics and Google Ads, BigQuery is perhaps the most popular data warehouse solution for marketing purposes.
- Amazon Redshift: Amazon’s high-capacity data warehouse runs on AWS and is popular among marketers thanks to its convenient integrations with popular BI tools such as Tableau, Yellowfin, and Power BI.
- Snowflake: Snowflake offers scalable and intuitive data warehousing solutions to the masses. With easy configuration, Snowflake removes the need for extra maintenance. Best of all, you can run it on top of your existing cloud infrastructure.
- Azure Synapse Analytics: Microsoft’s data warehousing platform offers petabyte-scale data warehousing. Microsoft offers a full ecosystem, using machine learning and Power BI natively inside Azure Synapse Analytics.
The good news is that in many companies, other departments like finance and/or sales are already using a data warehouse before marketing catches up. In these cases, the best course of action is often to simply start moving marketing data to the existing data warehouse solution.
Other common considerations include:
- Compatibility with current IT infrastructure
- Cost of implementation and storage
- Integrations with data sources
- Integrations with business intelligence and/or reporting tools
- Security
If you find yourself in a situation where you need to compare and recommend a data warehouse solution for marketing purposes, make sure to check out this comparison between Google BigQuery, Snowflake, and Redshift for marketing data warehousing.
7. How to get started with marketing data warehousing
Let’s say that you’ve chosen the data warehouse to which you want to start moving your marketing data.
Next, it’s time to roll up your sleeves and get down to business. That involves the following three steps:
- Marketing data warehouse design
- Data modeling and setting up data transfers
- Automating marketing dashboards
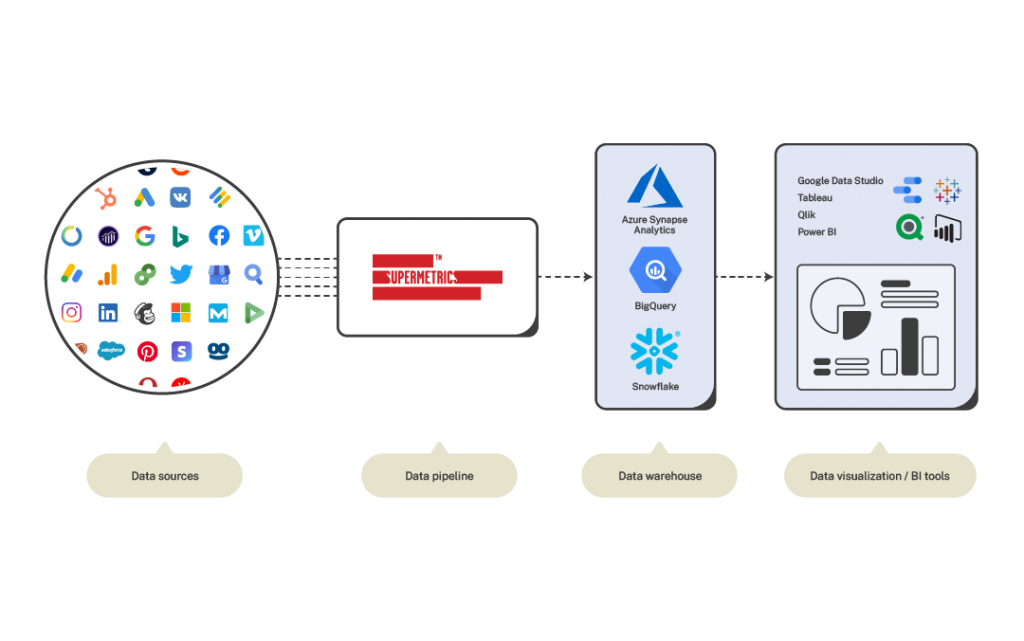
Let’s look at each of these in more detail:
A. Marketing data warehouse design
In simple terms, marketing data warehouse design consists of three steps:
- Identifying the data sources from which you want to pull data
- Deciding which data warehouse solution to move that data to (which we already discussed above)
- Choosing the analytics and reporting layer
First up, you’ll want to make a list of the data sources you’ll want to connect with your warehouse.
Common marketing data sources include:
- Digital advertising platforms such as Facebook, Google, LinkedIn, Snapchat, TikTok, Twitter, Criteo, Taboola, and Outbrain.
- Web analytics tools like Adobe Analytics and Google Analytics.
- CRM systems like Salesforce and HubSpot.
- Customer data platforms like Segment and Exponea.
- Marketing automation platforms such as HubSpot, Mailchimp, and Klaviyo.
- Ecommerce and payments platforms like Shopify, Stripe, and PayPal.
- Social media platforms such as Instagram, Twitter, LinkedIn, and Facebook.
When you know what data sources you’ll want to pull data from and to which data warehouse, it’s time to consider the last part of the puzzle: your reporting and analytics stack.
Common business intelligence, reporting, dashboard tools that integrate with data warehouses include:
- Google Data Studio
- Tableau
- Looker
- Qlik
- Power BI
These tools allow you to create automated dashboards that help the marketing team analyze full-funnel marketing performance and drill down to the performance of specific marketing channels such as advertising, email marketing, and content marketing.
When choosing the right reporting and analytics layer, it’s a good idea to consider at least the team’s skills and compatibility with the chosen data warehouse.
B. Data modeling and setting up data transfers
Once you’re done with all your high-level technology decisions, it’s time to move on to the nitty-gritty, i.e. data modeling and setting up data transfers into your data warehouse.
The first decision you’ll need to make here is whether you want to build your own data pipelines, hire an external vendor to build them for you, or use fully managed marketing data pipelines (like Supermetrics).
Once you’ve made that decision, it’s time to move on to data modeling, i.e. listing the actual metrics and dimensions you’ll want to move from each data source to your marketing data warehouse and deciding which schemas to use for each transfer. You also have to plan for the timeframe that you are backfilling your dataset with past data. The goal of data modeling is to make sure that the data you’ll store in your warehouse is as clean, consistent, and usable as possible.
Data warehouses organize data in structures called schemas. For your cross-channel data to be as comparable as possible, you’ll want to make sure to use consistent naming conventions and standard schemas whenever possible. However, data pipelines like Supermetrics also allow you to create custom schemas for less typical use cases.
Next up, it’s time to set up your data transfers. If you’ve decided to build (or outsource the building of) your own API connections, this may take anywhere between a couple of weeks and several months. With a data pipeline like Supermetrics, on the other hand, you can set up and automate your cross-channel data transfers in less than an hour.
Check out how easy it is to get your first transfers up and running with Supermetrics for BigQuery.
Psst! You can now get a free 14-day trial of Supermetrics for BigQuery or Supermetrics for Snowflake to see how easy it is to start moving your marketing data to a data warehouse.
C. Analysis and reporting
When you have your data flowing safely into your data warehouse, there’s only one thing left for you to do: building dashboards and reports to make the data accessible to the marketing team.
As discussed earlier in this article, only very few marketers know how to write SQL scripts to get the data they need out of the data warehouse. That’s why it’s a good idea to try to answer the marketing team’s most typical data needs with ready-made dashboards.
Pro tip: By including as many useful filters and dropdowns in your dashboards as possible, marketers can independently analyze the type of granular data they need for decision-making.
No number of dashboards will, however, save your analytics team from occasional ad hoc analytics. But as long as you can cover ~80% of the marketing team’s data needs with pre-built dashboards, the analytics team is unlikely to become a bottleneck.
8. Over to you
Congrats on finishing this beast of a post!
We genuinely hope that this post helped you find answers to any questions you may have had about marketing data warehousing.
But if you’re still not quite sure how to get started or if you’d like to hear more about how Supermetrics can help, our team is here for you.
Don’t forget that you can always start a free 14-day trial of any Supermetrics product.
Turn your marketing data into opportunity
We streamline your marketing data so you can focus on the insights.