Nov 2, 2023
Marketing mix modeling for marketers: How to measure marketing in a privacy-first world
12-MINUTE READ | By Zach Bricker
[ Updated Feb 2, 2024 ]
As we’re transitioning to a privacy-first digital marketing world, marketers need to move away from tracking methods relying on clicks, such as multi-touch attribution.
Marketing mix modeling (MMM) isn’t a new technology for measuring marketing. And thanks to automation, it’s a more efficient and accessible tool that can help businesses maximize their marketing ROI without relying on user-level data.
In this post, we’ll discuss MMM—what it is, what you can do with it, and some tips and resources to get started.
In this article:
- What is marketing mix modeling
- What you can do with marketing mix modeling
- What types of data are needed for marketing mix modeling
- How do you know if your MMM is accurate
- Marketing mix modeling has become more accessible
- The future of marketing mix modeling
- Marketing mix modeling vs. multi-touch attribution
- How to get started with marketing mix modeling
- 3 best practices for doing marketing mix modeling
- Additional learning resources
What is marketing mix modeling (mmm)?
Marketing mix modeling is an analysis technique for determining which marketing efforts drive sales most effectively. It looks at past data to see what worked and what didn’t, and then it helps you plan future campaigns that are more likely to succeed. By using MMM, you can better use your marketing budget and get more value from it.
MMM helps you predict the impact of different marketing strategies and scenarios, allowing you to make better budget decisions and optimize your marketing spend.
What can you do with marketing mix modeling?
We’ve been talking about the theory for quite a bit. Now, let’s look at some examples of what you can do with a marketing mix model. Typically, with MMM, businesses can understand marketing effectiveness by:
- Measuring incremental sales
- Know which marketing channels bring you more base sales
- Optimize budgets and sales by forecasting
Measuring incremental sales
In a marketing mix model, sales can be divided into:
- Base sales are sales that happen regardless of marketing activities, for example, returning customers. Brand effects or external factors like economic conditions can affect your base sales.
- Incremental sales are sales that are driven by marketing activities. For example, paid ads.
In a webinar about building MMM we did with dentsu Norway, the dentsu team showed an example to demonstrate the results from their marketing mix model. One use case was how much of the total sales is base sales and how much of it is incremental sales. This helped them understand how each channel has contributed to driving sales and figure out which marketing channels give the best bang for the buck.

Know which marketing channels bring you more base sales
Brand activities can positively influence your base sales. When you’re running ads on TV, radio, or social media, it’s possible that the audience won’t convert immediately. But that doesn’t mean your marketing campaigns fail. You can still succeed in building awareness, so whenever the audience is ready to purchase, your brand will be at the top of their mind.
The marketing mix model can help you measure the brand awareness and ad recall effects. It helps separate the short-term and long-term effects of different marketing channels. For example, TV or out-of-home (OOH) advertising are usually channels that drive results in a longer term compared to channels like Google Ads.
By mapping out the effects of different channels, you can also spot marketing campaigns that don’t have any value, stop spending the budget on those, and allocate the budget where you see the best results on marketing performance.

Watch the dentsu Norway team explain the short and long-term effects of different channels >>
Optimize budgets and sales by forecasting
Every marketing campaign will reach a threshold where each additional dollar you put in will drive lower impacts. This is called the diminishing return effect. A marketing mix model (MMM) can help you identify your point of diminishing returns, which allows you to improve your strategy to get the most optimal results.
For example, you can run different scenarios to see how your sales will change based on different budget allocations. This will help you manage your marketing budget effectively.
What types of data are typically used in a marketing mix model
When doing MMM, it’s important to include:
- Sales data, for example, historical sales data, revenue, unit shares, market share, etc.
- Media spend data, for example, your spend on different channels, including digital and offline channels.
Besides these two primary types of data, you should also incorporate other aggregated data sources such as:
- Competitive data, for example, marketing activities of your industry or competitor benchmarking data, etc.
- Macroeconomic data, for example, GDP growth or unemployment rates, etc.
- Consumer data, for example, demographics, interests, behaviors, etc.
These data sources serve as the foundation for the analysis. By using a variety of data sources, the marketing mix model can provide a comprehensive understanding of the impact of marketing tactics on sales.
How do you know if your marketing mix model is reliable?
Now comes one of the most important questions: “How can I trust the results of my marketing mix model?”
There are different ways you can use to validate the accuracy and reliability of the marketing mix model. One common method is to compare the model’s predictions to actual sales data and business outcomes. But, the ultimate validation of MMM results is through real-world incremental experiments, such as hold-out forecasting, conversion lift studies, etc. You can conduct these experiments in the ad platforms. For example, Meta has its own conversion lift study tool, which can help minimize bias and ensure the accuracy and reliability of MMM models.
Marketing mix modeling has become more accessible
Marketing mix modeling isn’t a new measurement approach. In fact, it dates back to the 1980s when Procter & Gamble began deploying a system of analytics to measure their marketing efforts. It has been popular among brands across categories like CPG and retail since their customer touchpoints are heavily divided between online and offline, making reliable attribution difficult.
Before, MMM was quite slow, manual, and expensive to do. It required a lot of resources to collect, clean, and prep the data. For example, it took up to 30 to 60 days to get data from TV commercials, radio ads, or billboards.
Luckily, nowadays, there are many tools that help automate MMM, which makes it faster, more efficient, and more accessible. For example, if you’re just starting out and you don’t have enormous datasets yet, you can even automate your marketing mix model using Google Sheets.
As a result, MMM is becoming more popular among other industries, such as gaming advertisers, digital natives, and omnichannel companies.
The future of marketing mix modeling
Compared to the traditional MMM, new technology, AI, and machine learning have changed the way MMM works and will continue to influence the next generation of MMM. To name a few shifts:
- The increasing use of AI and machine learning: Large organizations are creating advanced models like LLMs (Large Language Models) such as Chat GPT and Bard. As these models mature, they will play a more prominent role in improving the accuracy and actionability of MMM results.
- The expansion of MMM to new industries: MMM is no longer just limited to the consumer goods industry. It is now being used by businesses in a wide range of industries, including healthcare, financial services, and technology.
- The rise of real-time MMM: Real-time marketing mix modeling allows businesses to get insights into their marketing performance more quickly, which can help them make better data-driven decisions about their marketing campaigns.
Marketing mix modeling vs. multi-touch attribution
While marketing mix modeling and attribution modeling can both be used to better understand the return on marketing investment, there’s a fundamental difference between the two concepts.
Attribution modeling boils down to assigning a specific dollar value to each individual marketing and/or sales touchpoint in or near real-time. MMM, on the other hand, is used to aggregate (a combination of internal and external) historical data to arrive at macro-level conclusions about the effectiveness of different marketing strategies.
As many institutions have rolled out privacy regulations to protect their citizens’ privacy and limit the data businesses can access, it’s getting harder to do multi-touch attribution without third-party cookies. That’s why more businesses start investing in MMM to better understand which growth levers to pull based on historical performance data for ideal business outcomes.
How to get started with marketing mix modeling
If you’re eager to get started with marketing mix modeling, you can follow the following steps:
- Get executives’ buy-in
- Define the business questions you want to answer
- Collect your data
- Run and iterate your model
- Use the insights to make decisions
Get executives’ buy-in
Implementing MMM for the first time is a significant decision. That’s why adopting MMM should be a top-down decision. Businesses should set a three-year plan to evaluate their options carefully. For example, the decision to do MMM in-house or work with a trusted provider depends on your goals, vision, and available talent. In-housing is feasible but requires a dedicated team of experts. In that case, you can consider collaborating with a trusted MMM provider.
The key is to align your approach with your specific goals and resources. We recommend marketing leaders work with data leaders in your organization to iron out the details and agree on the best approach to MMM.
Define the business questions you want to answer
The results of your MMM efforts will only ever be as good as the questions you ask. And that’s why the first thing you must do is define the questions you want to answer.
Here are a few examples of the questions you can ask:
- How does our brand’s social media engagement correlate with sales?
- How do our Facebook Ads correlate with brand awareness?
- What drives retention?
- Which marketing channels are most effective?
- How do market changes or competitor actions impact our sales and marketing results?
- How does pricing affect our marketing results?
- Are the returns of our marketing spend diminishing? Should we allocate our budget elsewhere, and if so, where?
Collect your data
The best models we see include detailed variables such as digital marketing tactics and optimization, granular formats, different types of advertising, customer touchpoints, etc.
Let’s say you want to answer the first question: “How does our brand’s social media engagement correlate with sales?” you’d need to pull:
- Social media engagement data from platforms like Facebook, Instagram, TikTok, Snapchat, LinkedIn, and/or Twitter.
- Revenue data.
You can use a data platform like Supermetrics to automate data collection and manage your data quality before feeding it into your marketing mix model.
The key is having a data architecture and strategy that can support MMM, you can build into that a grade of data that powers as many variables as needed.
Run and iterate the model
Once you have your data ready, you can feed it into your model. Depending on the complexity of your question, you may need to test the model with different data sources, metrics, and dimensions.
It’s important to keep testing and calculate the confidence level of the model and tweak and iterate it until you get the desired results.
Watch dentsu Norway walk through an example of a marketing mix model setup >>
Use the insights to make decisions
Data and insights mean nothing if you don’t use them to make decisions and improve your performance. Use the results from your model to inform your next actions, optimize your budget allocation, and improve marketing efficiency.
3 best practices for doing marketing mix modeling
Building a successful MMM strategy requires a well-rounded approach that takes into account data quality, expertise, and continuous learning.
Start small and iterate
Starting small is a prudent approach when implementing MMM. Begin with a limited scope to test its viability for your organization.
Make sure your data is clean and comprehensive
Ensure that your data is clean and comprehensive. The quality of your results heavily depends on the quality of the data you provide. You won’t get a good model if your data is bad. That’s why it’s important to follow good marketing data governance practices to make sure that your data is good and ready for marketing mix modeling.
Collaborate with experts in the field
Collaborate with experts in the field, whether it’s hiring an expert, engaging an agency, or partnering with specialists. Integrate insights from subject matter experts to combine the data-driven results with domain expertise.
Never stop learning
MMM and marketing measurement keep evolving, so it’s important to stay up to date and educate yourself on different measurement methods and new technologies. In case you want to dive deeper into MMM, we have some useful resources for you.
If you’d like to learn more about it at a high level, check out these non-technical resources:
- An Analyst’s Guide to MMM, published by Meta.
- The MMM Handbook by Google is a quick 7-page read that can help you start to contextualize the conversation in your organization about how, why, and who you should partner with
- Build Trust in Marketing Mix Modeling Across your Organization, published by Gartner, is a great asset in how to shed some of the old suppositions about MMM and show thoughtfully how the market has changed and can support the models.
- How dentsu Norway built marketing mix models using BigQuery and Supermetrics. This post provides a high-level overview of how dentsu, a global marketing agency, plans and rethinks its data strategy to build marketing mix models for its clients.
If you want to start building your own model, these resources are for you:
- An Introduction to Statistical Learning: with Applications in R by James, Witten, Hastie, and Tibshirani
- The Elements of Statistical Learning: Data Mining, Inference, and Prediction by Hastie, Tibshirani, and Friedman
After the basis, you can move into the more specific spaces of marketing research and analysis. Check out these books:
- Understanding Advertising Adstock Transformations by Joseph
- R /Python for Marketing Analytics and Research by Chapman
Want to take your marketing data to the next level?
Learn about Supermetrics' best-in-class marketing data platform, which puts you back in the driver's seat of your marketing budget.
About the author
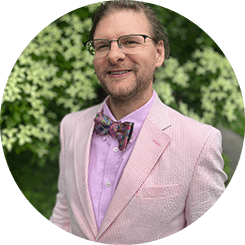
Zach Bricker
Zach leads the Solutions Engineer team at Supermetrics in the US. In his role, he's helping brands improve their marketing analytics, MTA, and MMM capabilities.
Stay in the loop with our newsletter
Be the first to hear about product updates and marketing data tips