Nov 4, 2022
Marketing mix modeling vs attribution: the pros and cons
10-MINUTE READ | By Evan Kaeding and Pinja Dodik
[ Updated Jan 31, 2024 ]
Most marketing teams have some kind of data problem.
Either they don’t have enough data, they have too much of it, or most commonly, they don’t know what to do with it.
And that’s why in this post, we’ll evaluate two of the most common ways businesses are currently putting their marketing data to work: attribution modeling and marketing mix modeling.
Skip ahead >>
Let’s dive right in.
What is attribution modeling?
Attribution modeling is the process of setting rules that determine how much each touchpoint along the customer journey contributes to a sale. This is done to estimate the revenue contribution of marketing.
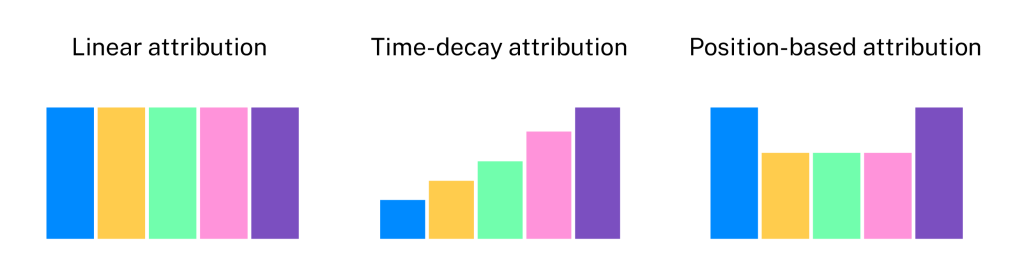
Common multi-touch attribution models include:
- Linear attribution: each (trackable) touchpoint along the customer journey has equal revenue contribution.
- Time-decay attribution: more recent touchpoints along the customer journey contribute to revenue more than earlier touchpoints.
- Position-based attribution: the first and last touchpoints contribute to revenue more than the touchpoints in the middle of the customer journey.
The benefits of attribution modeling
On paper, the promise of attribution modeling is attractive: it helps marketers dissect the customer journey and understand how each touchpoint contributes to a sale. Theoretically, attribution models should help marketers allocate their budgets more effectively to the channels, campaigns, and activities that have the largest impact on revenue.
However, the keyword here is ‘theoretically’. Let’s look at some of the reasons why attribution modeling often fails on its promise.
The drawbacks of attribution modeling
1. The false promise of 100% trackability
Let’s start with the biggest issue: multi-touch attribution is built on a false promise that every single touchpoint on every single device is trackable.
The problem is, that promise was made in bad faith.
We simply never were able to track every person’s every impression and click. And for legal, ethical, and technical reasons, we’ll never be able to either. In fact, the death of the cookie and the introduction of iOS 14 actually mean that the number of trackable touchpoints is declining, making attribution even more difficult.
Sidebar: The proponents of multi-touch attribution would argue here that collecting data about 70% of customer touchpoints is better than collecting 0% of that data. The problem with this argument is that no matter how you slice it, you’ll end up overvaluing the channels you can, in fact, measure. And as a result, you might end up making some pretty terrible decisions that ignore the most important (?) 30% of your customer journey.
What should you do with the data you’re collecting, then? Well, we’ll get to that later.
2. Attribution measures clicks—and clicks alone
The reason why attribution fails to account for many of the touchpoints along the customer journey is that attribution models typically measure clicks, and clicks alone. In other words, any offline touchpoints, dark social, and impressions are entirely left out of most models.
That podcast that had your CEO as a guest? Unattributable. That LinkedIn post that didn’t link to your website? Unattributable. That Slack group where someone recommended your product? Also unattributable.
Knowing this and having a general understanding of how people make decisions, do you think it’s okay that we’re including our Facebook and Google Ads, landing page visits, and webinar sign-ups in our attribution models—but failing to account for personal recommendations from peers, podcasts, and social media posts?
3. Attribution is, in fact, completely made up
These two points bring us neatly to our third one: attribution is made up.
As Tim Wilson points out in an interview on the Marketing Analytics Show, “the way we do attribution is laughable to data scientists. It’s absolutely ridiculous to be picking a heuristic model of last-touch, first-touch, time-decay, or inverse J-curve. And that’s for two reasons: first, it’s really hard to track one person across all touchpoints, and second, attribution also ignores a person’s history with the brand.”
Scientifically speaking, how would you prove that a certain click had a positive — or any — impact on a buying decision? Or that one touchpoint had a larger contribution than another? When you think about it, you’ll realize that the way we’ve decided to allocate credit to each touchpoint isn’t based on any science at all.
In fact, in most cases, it could be argued that people were going to buy our product regardless of (or despite) our ads. Yet, the ads will claim some credit for the purchase because, technically, they were shown to the customer before they made the purchase.
4. Good vibes only
Finally, most attribution models assume that every touchpoint has a positive impact on the buying decision. That’s problematic, considering that people can end up buying products or services with which they’ve had negative interactions.
In other words, multi-touch attribution can be considered the data equivalent to toxic positivity.
What is marketing mix modeling?
Marketing mix modeling refers to statistical analysis of historical marketing and sales data to estimate the impact specific future marketing activities will have on revenue—or any other dependent variable. This is done to optimize the allocation of the marketing budget to the right channels and campaigns.
The benefits of marketing mix modeling
Unlike multi-touch attribution, marketing mix modeling focuses on analyzing the inputs (think budgets and channels) in relation to the outputs (think revenue or brand awareness), using statistical methods instead of a weak chain of trackable customer actions.
In other words, the goal isn’t to build a comprehensive model of the customer journey, but rather to understand the role each channel and campaign plays in the overall success of the business.
What’s more, marketing mix modeling is based on real and valid science. MMM is applied linear regression, which can be far easier to measure reliably because all the inputs are known.
Finally, unlike MTA, MMM will also account for the negative revenue impact of certain channels, campaigns, or assets.
Sounds great on paper, right? However, that doesn’t mean marketing mix modeling doesn’t have its own issues.
The drawbacks of marketing mix modeling
1. For marketing mix modeling to work, you need a relatively large budget
Perhaps the biggest issue with marketing mix modeling is that it only starts making sense when your company reaches a certain scale — and media budget. This is because you need to create variability in your marketing mix to really start seeing the impact of each channel and campaign.
For example, if you’re only spending $1,000 a month on ads, it’s unlikely that you’ll see much of an effect, regardless of how you split the budget between different channels, ad types, and creatives.
2. MMMs typically ignore the counterfactual
The other big issue with traditional marketing mix modeling is that it ignores the counterfactual. In other words, it’s not common to include control groups or incrementality tests in marketing mix modeling.
Instead, most marketing mix models focus on the global population (in the statistical sense), which makes it difficult to understand the true impact of the changes you’re making to your budget allocation and channel mix.
However, there is nothing in the rulebook that says you can’t combine MMM with randomized control studies to make your findings more reliable.
3. Over-emphasis on conversions
In most cases, MMMs will underweight awareness-building activities at the top of the funnel. This is because most MMMs look at revenue as the dependent variable and spend as the independent variable.
In other words, if your input is marketing spend, your output can’t be brand awareness.
However, you can, of course, mitigate this issue by making brand awareness your dependent variable.
4. MMM gets tricky in a B2B environment
Much like multi-touch attribution, marketing mix models are also easier to implement in B2C environments than they are in B2B.
This is because MMMs assume that the population is homogeneous—and not composed of different roles and personas with unique goals, challenges, and priorities.
This, however, doesn’t mean that marketing mix modeling can’t work in a B2B setting. It just means that implementing it will be slightly trickier.
MMM vs. MTA: the final score
Long story short, marketing mix modeling is a far better solution for those who have the budget and means for it. At Supermetrics, we’ve already helped a number of clients implement a successful recipe for the cookieless world, as Fiksuruoka CMO Riku Poutanen puts it.
And if you don’t, you might be better off with simple A/B testing and conversion rate optimization than attribution modeling. That’s unless you can be relatively sure that your attribution model catches data on your most important touchpoints.
After all, sometimes acting based on limited data is better than building a complex model that only gives you a fraction of the truth.
About the authors
Pinja is a demand gen marketer with almost a decade of experience in B2B brand, content, and product marketing in the SaaS, tech, and agency world. She now leads marketing at Swarmia.
Evan is the Lead Solutions Engineer at Supermetrics. Thanks to his lengthy background in data and analytics engineering, he is a huge advocate for building better data stacks for businesses around the world.
Pinja and Evan debunk myths about analytics and attribution and help businesses get better at using data.
Turn your marketing data into opportunity
We streamline your marketing data so you can focus on the insights.