Jun 13, 2024
Incrementality testing for marketers
10-MINUTE READ | By Olivia Kory & Erkki Paunonen
[ Updated Jun 13, 2024 ]
In marketing, we often rely heavily on metrics that indicate direct conversions—whether it’s a purchase or an app download. But what about organic demand, word-of-mouth, or brand loyalty? How do they help drive the bottom-line numbers? And how can you separate them from paid activities?
Incrementality can help you answer those questions and understand the true marketing impact.
We’ll discuss what you need to know about incrementality testing, including:
- What’s incrementality in marketing
- Why do you need incrementality testing?
- How to get started with incrementality testing
- 3 marketing incrementality tests
- What kinds of companies can do incrementality testing
- What’s the difference between incrementality testing and A/B testing
- Do multi-touch attribution and marketing mix modeling measure incrementality?
You can also check out the podcast episode we did with Olivia about incrementality testing.
What’s incrementality in marketing?
Incrementality loosely means “causation.” It’s a test that helps you quantify the incremental impact of your marketing activities. It answers, “How many conversions are we getting with each additional dollar?”
Incrementality isn’t a new concept. In fact, it’s widely used in medical experiments. For example, in a drug trial, researchers don’t just administer the drug and observe the results. Instead, they establish two groups—one that receives the drug and one that does not. By comparing the outcomes between these two groups, researchers can gauge the efficacy of the drug.
Similarly, you can run a marketing experiment to understand the impact of marketing. Let’s say you’re testing the effectiveness of an ad campaign. You want to understand:
- Was it truly the ads that drove revenue?
- Would we have made the same revenue without the ads?
One way to do this is to run ads in some % of the United States and turn off the ads in the rest. Then, you can compare the results and determine the impact of your ads.

Why do you need incrementality testing?
Isolate the effects of marketing efforts from word-of-mouth and other factors
For well-established brands, where organic demand and word-of-mouth play significant roles, untangling the impact of advertising becomes exceedingly challenging.
Let’s take the 2023 box office hit Barbie promotion as an example. While the marketing efforts were lauded as genius, if you think about it, how much of the audience would’ve watched the movie regardless, e.g., with only a simple reminder of the release date?
Barbie is a household name, so many people may have seen the movie anyway because of their existing awareness and connection to the Barbie brand.
This inherent brand awareness makes it difficult to assess the actual impact of paid ads on consumer behavior.
Click-based attribution can mislead investments
One of the most important reasons for incrementality testing is to better manage marketing budgets.
Click-based attribution might overestimate the influence of ads. For example:
- Brand search: brand search can look fantastic in a click-based attribution model. when a user searches for a brand and clicks on an ad, it’s easy to attribute the subsequent action to the ad. In reality, if someone is searching for your brand, they’re expressing an intent and would likely visit your website and make a purchase anyway.
- Retargeting: if a user has already shown intent by visiting a website, bombarding them with retargeting ads may not significantly influence their decision-making process. Yet, in a click-based attribution model, these ads may appear highly effective, leading to continued investment in the channel.
How to get started with incrementality
There are several incrementality tests and tools you can use. But before rolling your sleeves and committing to anything, you need to do some groundwork. Here are some practical steps we follow at Haus to make sure we run a successful test.
1. Get your organization aligned
To get started, you should set expectations and establish a baseline understanding of incrementality in your team. It’s especially important to define what incrementality is measuring: the real impact of marketing efforts for better decision-making.
Challenge: Most of the time, incrementality is rejected because it isn’t understood. To avoid this, explain how this approach differs from the status quo so they don’t reject the results once they see them.
Our recommendation: Educate your team on the differences between the incrementality approach and the way things were done before. Emphasize the value and ROI this approach can bring. Make sure you have buy-in from the executive level. To set a clear course of action and scope your testing, build a roadmap for the first 30, 60, and 90 days defining your testing priorities.
2. Consolidate and normalize your data
Before beginning this step, you might want to conduct data quality audits. Some experiments, like Geo testing, require 6-12 months of sales data with geographic tags and ad spend from the same period to segment regions, so it’s worth it to take stock of your data.
Once you have aligned with a business case and know the state of your data, consolidate your ad spend data, conversion data, and platform data in a centralized view.
Challenge: If you don’t have your data in one place, it can get really messy. For some companies, siloed ad spend and sales data are the biggest roadblocks to incrementality.
Our recommendation: Make sure that the data you use for testing is your source of truth – If you’re using sales data, ensure it’s accurate and continuously validate that. Also, to set up a marketing data integration combining data from all your sources, consider a solution like Supermetrics and reinforce your marketing data governance policies.
3. Integrate your learning into day-to-day reporting
The best use case for incrementality findings is enriching existing measurement systems to provide a more accurate picture of marketing performance.
For example, if a test revealed that 50% of conversions reported by a platform were incremental, marketers could adjust their platform-reported conversions accordingly (e.g., by doubling the CPL/CPA figures). This approach allows for more accurate reporting and provides a realistic view of daily performance. But to go to the ‘next level’ and calibrate your future performance data with incrementality factors regularly, i.e., see your iCPAs day over day,
Challenge: Make sure you aren’t applying your incrementality factors too broadly. For example, your brand search incrementality factor shouldn’t apply to all your Google tactics.
Our recommendation: Re-test your incrementality figures to keep them relevant. We recommend testing your big bets quarterly or every 3-6 months.
There are several incrementality tests and tools you can use. But before rolling your sleeves and committing to anything, you need to do some groundwork. Here are some practical steps we follow at Haus to ensure we run a successful test.
3 marketing incrementality tests and their pros and cons

1. Ad platform conversion lift studies
Conversion lift studies are the go-to incrementality test, but they have downsides. This method involves using a platform like Meta or Google to segment the user base into treatment and control groups so you can test the relative impact of a marketing effort. While effective, this method has faced challenges due to privacy changes and lack of standardization across platforms.
How it works: Ad platforms like Meta or Google will offer an automated incrementality test. The test compares treatment (test) and holdout (control) groups. While the treatment receives one marketing promotion, offer, or message, the holdout receives nothing. The holdout is usually affected by word-of-mouth or organic awareness (the free kind of brand noise), while the treatment gets the ‘marketing.’ At the end of the campaign, the lift in conversions between the two groups is measured to determine the incremental impact of the ads.
Pros:
- This method is very straightforward and automated, meaning less work for you.
- You don’t need a team of data scientists or any outside data. It all exists on the same platform.
Cons:
- You don’t know anything about the users.
- There is no standardized method across platforms.
- Due to the end of third-party cookies, you can no longer be certain that Google/Meta can track these users throughout the campaign’s lifetime.
- Platforms may restrict access to the test, e.g., based on your ad spend budget.
2. Geo testing
Geo-testing uses geographic regions to separate the treatment and holdout groups. Aggregate sales data from both groups are compared to assess the incremental impact of the ads. This method is one of our specialties at Haus and can provide insights for any type of channel (including offline) without messy user-level data issues.
How it works: A geo is evenly divided into treatment and control groups, with ads targeting only the treatment group. Then, like in a conversion lift study, one group (the treatment) receives the ads, while the holdout does not. Through this test, you can see the incremental impact of any marketing effort. The better your data, the more confident you can be in the test’s results. You need to work carefully with your data to ensure you can be confident in the accuracy of the geo split.
Pros:
- This is privacy-safe, meaning it doesn’t require any user data.
- You can do this test in any channel, online or offline.
- It’s a low barrier-to-entry method that’s great for young brands, e.g., when releasing new products.
Cons:
- It requires more ad spend because you’re looking at aggregates.
- It’s very resource-intensive to do on your own, so the complexity can require a data analyst when you don’t have a dedicated partner or solution.
3. Observational/ natural experiments
This basic approach measures the impact of a marketing change without a designated control group. As in previous examples, this test is like turning a light switch on and off: What happened before and after you made any marketing effort?
How it works: This experiment generally compares before and after a change. For example, if you’re rolling out a promotion, you would measure the sales during the promotion vs. before or against another similar timeframe. It’s much less precise, so it’s often a fallback if you can’t run a more controlled experiment.
Pros:
- This test is suitable for smaller brands and budgets. Observational experiments aren’t suitable for big brands since it’d be easier to see the topline impacts if the changes are big.
- It’s very simple to do and doesn’t require any intense data and analysis.
Cons:
- Unlike the first two testing methods, observational studies don’t have a real control group, so you’re never fully sure if external factors are muddying the data.
What kind of companies can do incrementality testing?
Incrementality testing is a solid fit for most company types. We at Haus have worked with brands of all sizes. But, as a rule of thumb, if you spend less than 5 million dollars a year on paid media, you probably don’t need intensive geo or platform lift incrementality testing. For smaller companies, observational experiments are a solid choice for getting a rough estimate of incrementality.
But, if you’re spending over 5 million, you’re likely generating more demand. The more word-of-mouth, organic demand, and PR noise you have, the harder it is to know the true impact of any particular channel. Or, if you have high-volume sales and plenty of geo-specific data, incrementality testing is a good option.
What kind of data do you need?
To perform an incrementality test, you need the outcome data, or in other words, the thing you’re trying to optimize for. So, if you’re an ecommerce company testing the impact of a campaign on revenue, you might need your revenue data.
Next, you need a way to divide the data into treatment and control groups. If you’re running a platform lift study, you’d need to provide the platform with your sales data so they can break it down by users. For Geo testing, you would need revenue by region.
So, the data you need is quite simply the event or actions you want to measure your test against, for example, revenue, app downloads, etc.
Does this work for B2B?
B2B attribution tends to suffer from long and complex sales cycles. This can reduce the data available to tie ad performance to sales, making incrementality testing tricky. In particular, low-volume, high-value purchases don’t provide enough sales data and require much longer testing periods to work.
But incrementality testing can still be advantageous. For instance, it can be used to test shallower KPIs like leads generated, or you can run a longer test (a 6-8 week experiment). As long as you’re interested in the KPI, it’s worth testing.
What’s the difference between incrementality testing and A/B testing?
An incrementality test is a kind of A/B test in which the B cell is a control group that gets no media at all. This allows you to establish a counterfactual to understand what would’ve happened without intervening.
While both incrementality testing and A/B testing are methodologies used to measure the effectiveness of marketing efforts, they differ in their scope, objective, and methodology:
- Scope: A/B testing focuses on optimizing individual elements or variants, while incrementality testing evaluates the overall impact of marketing efforts.
- Objective: A/B testing aims to identify the best-performing variant, whereas incrementality testing seeks to measure the incremental effect of marketing activities.
- Methodology: A/B testing involves randomized experiments with predefined variants, while incrementality testing employs experimental designs to isolate causal effects.
So, while A/B testing can be used to optimize specific elements of a campaign (like CTAs or subject lines), incrementality testing helps marketers make strategic decisions about resource allocation, channel optimization, and campaign effectiveness.

Do multi-touch attribution (MTA) and marketing mix modeling (MMM) measure incrementality?
Multi-touch attribution (MTA) and marketing mix modeling (MMM) are two commonly used marketing measurements that aim to attribute value to various marketing channels and tactics.
MTA is a method for attributing value to multiple touchpoints along the customer journey. Unlike traditional attribution models that assign credit to a single touchpoint (such as last-click attribution), MTA recognizes the influence of multiple interactions in driving conversions or other desired outcomes. But, MTA does not isolate the causal effect of marketing efforts from other factors. It can’t tell you whether the intended action would’ve happened anyway, as incrementality tests can.
Marketing Mix Modeling (MMM) measures impact in a big-picture way. It’s a statistical technique used to analyze the impact of various marketing activities on business outcomes. It helps you understand the incremental impact of marketing by showing how much each marketing input contributes to sales. You’ll see the combined impacts of marketing and non-marketing factors on your strategic KPIs.
Some final tips
We believe incrementality testing represents a powerful tool for marketers seeking to understand and optimize the impact of their advertising efforts. We’ll leave you with some final tips:
- Never stop testing: Incrementality testing is a tool in your kit that you should use alongside other marketing experiments to get closer to the truth.
- Apply your learnings to performance data: Incrementality factors can give you a more realistic picture of your marketing performance. Use them as qualifiers for daily KPIs to get clearer views of your channel performance.
- Use your findings to inform budgeting: Once you’ve identified the real impact of your marketing, you can allocate your ad spend and justify your marketing budget.
- Keep an open mind: You’re going to be continuously learning and challenging a lot of long-held assumptions.
To continue your learning, we suggest you read our guide on marketing measurement to identify further opportunities to layer multiple methodologies together.
Marketing measurement guide for marketers
In this post, we’ll discuss marketing measurement, including metrics, methods, tools, and especially how you can measure incrementality.
About the authors
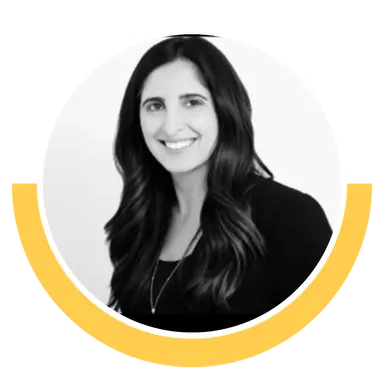
Olivia Kory
Olivia is the Head of Go-To-Market at Haus, an innovative incrementality and experimentation platform. With a deep-seated passion for performance marketing, Olivia has honed her skills over a remarkable career, leading growth at industry giants such as Sonos, Quibi, and Netflix. Her extensive experience in tackling complex marketing challenges inspired her journey at Haus, where she plays a pivotal role in driving the company's mission to empower businesses with cutting-edge solutions. Olivia's expertise and insights are invaluable to Haus's continued success in transforming the marketing landscape.
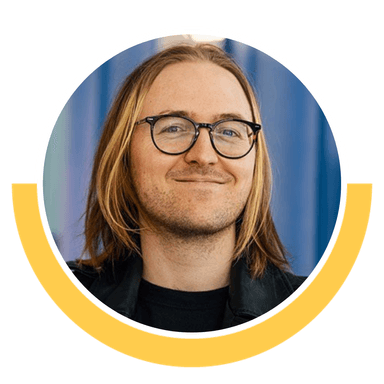
Erkki Paunonen
Erkki is a content and demand gen marketing pro with expertise in B2B SaaS. He creates revenue-generating marketing programs and writes non-boring content about MarTech and Procurement Analytics. Erkki lives with his partner, cat, and dog in Helsinki, where he enjoys Finland's renowned metal scene.
Stay in the loop with our newsletter
Be the first to hear about product updates and marketing data tips