Nov 18, 2022
Analytics maturity model: how to get the most out of your data strategy
12-MINUTE READ | By Pieter van Groenendael
[ Updated Oct 31, 2023 ]
Most marketers agree that non-cookie measurement methods will have a big impact on advertising. But, implementing these methods requires a certain level of analytics capability and access to the right data.
You might find yourself asking:
- What’s my current level of analytics capability?
- What can I learn about my business with it?
Keep reading to learn more about the analytics maturity model, how to figure out which step you’re at, and the best way to move forward.

Skip ahead >>
- What’s the analytics maturity model
- What are the different stages
- Where are you in the model
- How to choose the right model for you
What’s the analytics maturity model?
The analytics maturity model is a framework that helps businesses determine how they can use their data to find insights and make decisions rather than just collecting data for the sake of it.
There are several analytics maturity models—some most used ones are:
- Gartner’s—Maturity Model for Data and Analytics
- SAS—Analytic Maturity Scorecard
- OECD—Analytics Maturity Model
- DAMM—Data Analytics Maturity Model for Associations
We’ll use the Gartner model as a baseline. We chose their model because it’s easy to understand, actionable and applicable across segments outside of marketing—like sales, finance, etc. Also, since many analytics maturity models are quite similar, so you can apply the steps, actions, and recommendations in this post, regardless of the model you’re using.
What are the different stages in the analytics maturity model?
The Gartner model has four stages, with the first stage requiring some basic analytics skills. We added one more stage to the model—unstructured—to cover businesses that don’t have any analytics skills. Then you have descriptive, diagnostic, predictive, and prescriptive analytics. We’ll go through each one separately and help you understand your phase and how to move forward.

Unstructured
Unstructured data analysis means you have no data strategy and don’t use analytics at all.

Let’s say that you’re in the business of selling footwear—basketball sneakers. You have no idea how interested people are in a particular sneaker and why they would be.
Before getting to descriptive analytics, you’re in the lagging or restrictive stage. For the most part, most businesses start here. So, if you’re waiting to take the leap, you’re not alone.
Descriptive
Descriptive analytics—what happened—is the simplest form of analytics and the foundation for more in-depth types. Descriptive analytics summarizes what happened or is happening by pulling trends from raw data and providing insight into what these trends mean.

Continuing our earlier example, descriptive analytics can tell you something like: this basketball sneaker has a seasonal increase in interest in August, September, and early October each year.
You can use data visualizations to communicate descriptive analysis because charts, graphs, and maps can show trends in data—as well as dips and spikes—in a clear, and easily understandable way.
At this stage, you can use spreadsheet tools like Google Sheets, Excel, and data visualization tools like Looker Studio (formerly Data Studio) or Power BI.
Diagnostic
Taking the analysis a step further with diagnostic analytics—why did this happen— includes comparing trends or movement, seeing if there are correlations between certain statistics, and determining cause-and-effect relationships where possible.

You may look at the demographic data about which group most commonly uses basketball sneakers and find out that they’re between the ages of 13 and 16. But, the customers who buy these sneakers tend to be between 30 and 50. Some customer survey data collected earlier reveals that one of the main reasons customers buy basketball sneakers is parents gift them to their teenagers. The increased sales in the late summer and early autumn months may be due to the basketball season starting that year.
Although you can do diagnostic analytics manually in tools like Excel and Google Sheets, we commonly see marketers use a BI tool like Looker Studio or Power BI to apply simple logic to compare certain statistics to one another.
Using a data warehouse is also a popular option as it allows you to gather data from different sources—paid media and organic social—into a single location before feeding it to a visualization tool. You can apply the above concepts across various use cases—examining marketing demand and explaining customer behavior.
Predictive
Predictive analytics—what might happen in the future— historical data to make predictions on future trends or results. You can do it manually or with machine-learning algorithms.

Predictive analytics can be used in marketing to forecast sales trends at different times of the year and plan campaigns accordingly. For instance, knowing that basketball sneaker interest and sales have spiked in August, September, and early October every year for the past decade could help you predict that the same trend will occur next year. Historical behavioral data can also help you predict a person’s likelihood of going from brand awareness to product purchase. So, if you know that a person usually interacts with five pieces of content before buying, you can plan your content accordingly. Storing historical data in a data warehouse, like BigQuery, can help you do predictive analytics manually.
Data modeling tools, like dbt, can help automate combining information from different sources into insight-ready tables. Predictive analytics can be further automated using a machine learning platform. Additionally, having a solid dataset with machine-learning capabilities provides a smooth transition to the prescriptive level.
Prescriptive
Finally, prescriptive analytics—what should we do next—takes into account all possible factors in a scenario and suggests actionable takeaways.

To complete the basketball sneaker example. Now that you know the basketball season is starting soon and the demand for sneakers will increase—based on your trend analysis—what should you do? You could run an A/B test with two ad creatives. One that targets your product end-users—the teenagers. And one that targets your buyers—the parents. The experiment results can help you figure out how to take advantage of this short timeframe even better.
While manual prescriptive analysis is doable with smaller data sets, you’ll get better results by using machine-learning algorithms when working with larger amounts of data to get an answer to “what’s the best thing to do next?”. Platforms like Google Cloud Vision AI or Vertex AI can help you deploy these machine learning models.
Where are you in the model, and how can you move forward?
Before you decide what to focus on, it’s important to understand where your organization is in different areas of the analytics maturity model. Based on where you are, you can plan how to move forward.
1. Assessment
First, assess your current analytics maturity level to find out what data you’re collecting and using. Answer the following questions:
- What are your current data sources?
- Who has access to that data?
- What tools are you using?
2. Availability
Here, you should figure out what other sources of data are available to you, including:
- Internal data sources: CRM, web analytics, customer feedback
- External data sources: ad platforms, public data, etc.
3. Prioritization
Next, consider the metrics that you monitor and the questions they answer. Prioritize the ones that give you actionable insights about “why” and “how” instead of focusing on metrics that only give information about “how many”.
4. People
Ensure that relevant stakeholders have access to relevant data. Implement agile practices to break down the silos and simplify data sharing across departments. Consider giving employees access to data, teaching them how to use it, and encouraging new ideas.
5. Tech
Invest in technology that can help you interpret available data and get value out of it. You should think about your team’s analytics skills and choose the tools that are suitable for them. For non-technical users, it’s worth going for data visualization tools with a user-friendly interface to make reports easy to understand.
On the other hand, if your team has some SQL skills, they’ll get more value from using a data warehouse.
Go through these five aspects and ask yourself the same questions each time you plan on moving forward through the model to know if you’re ready.

How to choose the right model for you
Where you should be in the analytics maturity model comes down to who uses the data and what decisions you expect to draw from it.
While you can get better insights and ROI from moving up the model, especially with predictive and prescriptive analytics, you need to watch out for a few things.
You need accurate data to train your AI and machine learning models—the better the datasets, the better the predictions. If your data is incomplete or has wrong information, it’ll hurt your results.
For example, if the age demographic data from the basketball sneaker buyers contains only data about the end user, the teenagers, and leaves out the information that parents are the actual buyers of these sneakers. Your prescriptive model won’t consider the parents as a group to market your product to, and most will likely advise you to market only to teenagers. The marketing team will probably invest in the wrong audience and miss out on the opportunity to drive sales.
In that case, it would make more sense to use a descriptive model—what happened—or a diagnostic model—why did it happen—and build up the quality in those datasets before you can drive well-informed predictions and decisions generated by machine learning and AI in the later stages.
Wrap-up
The analytics maturity model helps you make the most of your data and improve decision-making. Each step in the model will prepare you for the next one.
So you can start by using descriptive analytics to tell the story of what is happening, then build on that and find out why those things are happening with the help of diagnostic analytics.
Once you have access to the right data and it’s reliable, you can move to predictive analytics to spot trends. And finally, you can use those predictions to inform what you should do in the future with prescriptive analytics.
Don’t worry if you’re not at the prescriptive phase yet, moving through the model is more about assessing your current needs and resources, and making the most of those before scaling too quickly. There is no right or wrong place to be, just the right place for your team and your business.
About the author
Pieter is a Sales Engineer at Supermetrics. He works closely together with customers to identify opportunities for increased value return in their marketing data stack. Outside of working hours, he can usually be found shooting hoops on the basketball court.
About the author
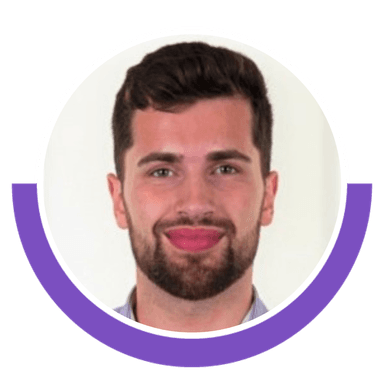
Pieter van Groenendael
Pieter is a Solutions Engineer at Supermetrics with over 10 years of experience in the data and analytics space. By sharing his first-hand experience and knowledge, he's helping hundreds of customers from different industries solve their data challenges. Besides, Pieter is an author on the Supermetrics blog, contributing various articles about data management and measurement.
Stay in the loop with our newsletter
Be the first to hear about product updates and marketing data tips