Sep 24, 2020
A better way to forecast the performance of your paid campaigns
5-MINUTE READ | By Taimur Abdaal
[ Updated Mar 9, 2023 ]
If French is the language of love, then metrics are the language of marketing.
CPCs, CPA, CTRs; no marketer can get by today without learning how to speak in terms of metrics. We use metrics for reporting data, forecasting performance, and for understanding how changes in the real world have impacted our marketing.
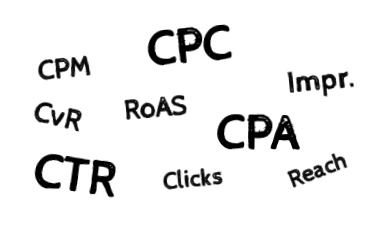
One thing that we tend to take for granted when we speak in terms of metrics is their certainty. We tend to speak of them as if they always have exact values.
This isn’t an issue when it comes to historical data, which is almost always certain. When it comes to demand forecasting though, we often forget that the future is far from certain.
Let’s take a look at the problems posed by ignoring uncertainty in our metrics, and how this hurts us when it comes to putting together forecasts.
At the end of the article, I’ve put together an interactive forecasting model that does account for uncertainty, which you can copy and use to forecast your own campaigns.
Why do we forecast the way we do?
We’re all guilty of making forecasts which state that our campaigns will generate this much revenue next quarter, and cost exactly that much.
When we make these forecasts, we know that they aren’t intended to be precisely accurate.
But if our forecasts aren’t supposed to be exact, then why do we make forecasts that output exact figures?
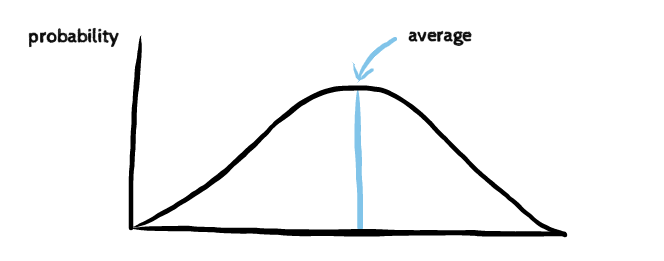
Often we just forecast an average value for metrics, ignoring the fact that there’s a whole range of values that metric could take.
One of the reasons that we tend to forecast exact figures is that it’s just plain difficult to build uncertainty in.
Uncertainty creeps in at every stage in the forecasting process. Say you’re trying to pull together a paid search forecast for next quarter, there’s a whole list of metrics that you won’t be able to predict with certainty:
- Search volume
- Impression share
- Click-through rate
- Cost per click
- Conversion rate
When all of these metrics carry their own amount of uncertainty with them, it can feel overwhelming to try and follow this uncertainty through into whatever final output (revenue, budget, etc.) you’re trying to forecast.
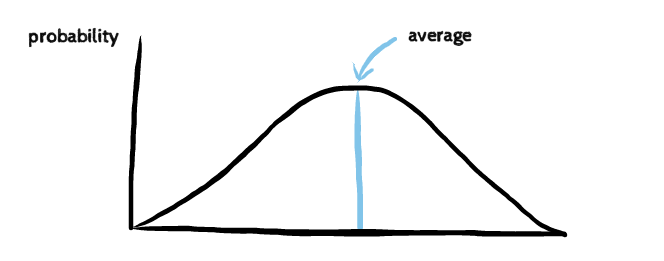
If each stage of the funnel introduces a small amount of uncertainty, this can multiply as you move down the funnel, making it difficult to forecast lower funnel metrics like conversions.
As a response to this, most of us take the easy way out. We pretend that we know what each of these metrics will be next quarter, and so we end up pretending we know exactly what all of our outputs will be.
The problem with exactness
Giving exact forecasts comes with issues though:
- If you’re providing the forecast to other stakeholders, and you claim that you’re able to generate a certain amount of volume next quarter, you can easily be held to whatever number you give. Given that you’re extremely unlikely to get your forecast perfectly correct, this can put unnecessary pressure on you.
- Stakeholders may need to make business decisions based on your forecasts. They might use your figures to inform stocking, hiring, or whatever other processes depend on marketing volumes. Giving exact forecasts for marketing volumes can make it difficult for these stakeholders to prepare for over or under-performance.
Accounting for uncertainty in forecasts helps solve both of these problems. Instead of outputting a single value for next quarter’s revenue, it lets you give a range of possible values that next quarter’s revenue will lie in.
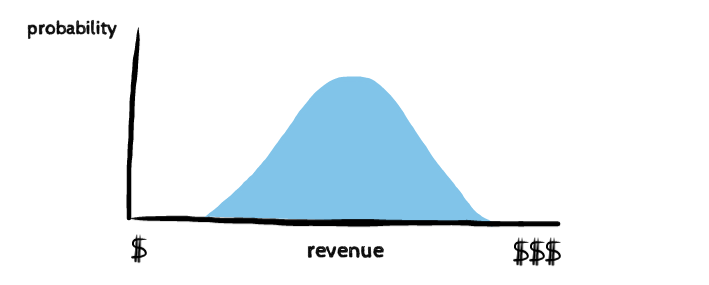
This takes the pressure off you; no longer are you going to be held to that one revenue number which your forecast spits out. It also lets other stakeholders plan for all possible scenarios based on how well your marketing goes, meaning you don’t have to stress over hitting your targets precisely.
So, how do you account for uncertainty?
Let’s run through a simple example to see how we can incorporate uncertainty into our forecasts.
Say we’re trying to forecast how many paid search impressions we’re going to get next quarter. We know that the number of impressions depends on how many searches there are, and our impression share.
We’re not going to think of next quarter’s number of searches and impression share as having fixed values. Instead, we’re going to think of them as ranges of values.
So, instead of saying that we expect to bid on 100k searches next quarter, we’ll say that we expect to bid on somewhere between 75k and 125k searches. Similarly, instead of a 70% impression share, we might expect to have an impression share between 60 and 80%.
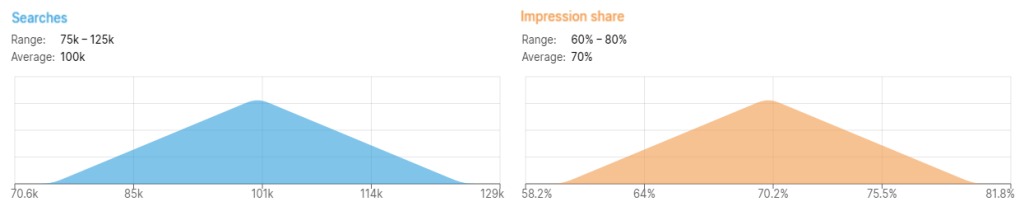
Giving ranges for these two metrics is all well and good, but how do we estimate the number of impressions we’ll get?
This is where simulations come in
We can run a large number of simulations, where in each simulation the number of searches is chosen randomly between the numbers 75k and 125k, and the impression share is chosen randomly between 60 and 80%. In each simulation we then multiply these two numbers together, and record the result; the number of impressions we receive in that simulation.
By running a large enough number of simulations, and getting enough estimates of the number of impressions, we can build up a distribution curve like the one below:
This curve tells us the probability that the number of impressions next quarter will be above or below any particular value.
An interactive forecasting tool
Interested in building your own forecasts that take care of uncertainty? I’ve put together an interactive calculator below, using a modeling tool called Causal.
Or open the interactive forecasting tool in a new tab >>
The calculator forecasts revenue from paid search campaigns. You can tweak the input assumptions to match your own campaigns, or you can click ‘Use this template’ to fully customize it.
Notice that inputs at the top can take ranges. Unsure what value a particular metric might take? Just write in the upper and lower bounds, and the model will take care of the rest.
The default values come from this sheet that uses Supermetrics for Google Sheets to pull historical data from Google Ads.
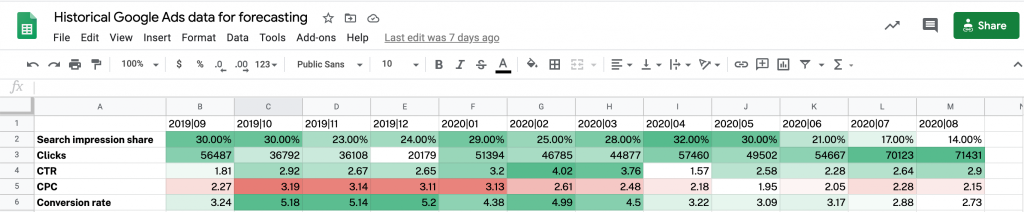
Editor’s note: You can use the Supermetrics add-on in Google Sheets (or Excel) to quickly pull all the historical data you need into a single spreadsheet tab. Then use the (monthly or weekly) min and max values as an input to Causal’s forecasting tool.
Happy forecasting! ?
Turn your marketing data into opportunity
We streamline your marketing data so you can focus on the insights.